Work in process
-
H. Lin, T.F.S., and F. Zaffora Blando:
Learning theory.
Invited by Oxford Bibliographies.
-
T.F.S.:
Values in machine learning: What follows from underdetermination? [philsci]
Submitted.
T.F.S.:
Statistical learning theory and Occam's razor: Structural risk minimization.
In process.
Peer-reviewed publications
-
T.F.S. (2025):
Statistical learning theory and Occam's razor: The core argument. [doi] [philsci] [arxiv] Minds and Machines 35, 3: 1-28.This paper distills the core argument for Occam's razor obtainable from statistical learning theory. The argument is that a simpler inductive model is better because it has better learning guarantees; however, the guarantees are model-relative and so the theoretical push for simplicity is checked by prior knowledge.
-
T.F.S. (2025):
On explaining the success of induction. [doi] [philsci]
The British Journal for the Philosophy of Science 76(1): 75-93.Douven (2021) observes that Schurz's meta-inductive justification of induction cannot explain the success of induction, and offers an explanation based on simulations of the social and evolutionary development of our inductive methods. I argue that this account does not answer the relevant explanatory question.
-
R.T. Stewart and T.F.S. (2022):
Peirce, pedigree, probability. [muse] [philpapers]
Transactions of the Charles S. Peirce Society 58(2): 138-166.One aspect of Peirce's thought is his rejection of pedigree epistemology, the concern with the justification of current beliefs. We argue that Peirce's well-known criticism of subjective probability is actually of this kind, and connect the question of pedigree to the contemporary debate about the Bayesian problem of the priors.
-
T.F.S. (2022):
On characterizations of learnability with computable learners. [pmlr] [arxiv]
Proceedings of Machine Learning Research 178 (COLT 2022): 3365-3379.I study computable PAC (CPAC) learning as introduced by Agarwal et al. (2020). I give a characterization of strong CPAC learning, and solve the open problem posed by Agarwal et al. (2021) on improper CPAC learnability. I further give a basic argument to exhibit undecidability of learnability, and initiate a study of the arithmetical complexity. I briefly discuss the relation to the undecidability result of Ben-David et al. (2019).
-
T.F.S. and R. de Heide (2022):
On the truth-convergence of open-minded Bayesianism. [doi] [philsci] The Review of Symbolic Logic 15(1): 64-100.Wenmackers and Romeijn (2016) develop an extension of Bayesian confirmation theory that can deal with newly proposed hypotheses. We demonstrate that their open-minded Bayesians do not preserve the classic guarantee of weak truth-merger, and advance a forward-looking open-minded Bayesian that does.
-
T.F.S. and P.D. Grünwald (2021):
The no-free-lunch theorems of supervised learning. [doi] [philsci] [arxiv]
Synthese 199: 9979-10015.The no-free-lunch theorems promote a skeptical conclusion that all learning algorithms equally lack justification. But how could this leave room for learning theory, that shows that some algorithms are better than others? We solve this puzzle by drawing a distinction between data-only and model-based algorithms.
-
T.F.S. (2020):
The meta-inductive justification of induction. [doi] [philsci] Episteme 17(4): 519-541.I discuss and reconstruct Schurz's proposed meta-inductive justification of induction, that is grounded in results from machine learning. I point out some qualifications, including that it can at most justify sticking with object-induction for now. I also explain how meta-induction is a generalization of Bayesian prediction.
-
T.F.S. (2019):
The meta-inductive justification of induction: The pool of strategies. [doi] [philsci] Philosophy of Science 86(5): 981-992.I pose a challenge to Schurz's proposed meta-inductive justification of induction. I argue that Schurz's argument requires a dynamic notion of optimality that can deal with an expanding pool of prediction strategies.
-
T.F.S. (2019):
Putnam's diagonal argument and the impossibility of a universal learning machine. [doi] [philsci] Erkenntnis 84(3): 633-656.The diagonalization argument of Putnam (1963) denies the possibility of a universal learning machine. Yet the proposal of Solomonoff (1964), made precise by Levin (1970), promises precisely such a thing. In this paper I discuss how this proposal is designed to evade diagonalization, but still falls prey to it.
-
T.F.S. (2017):
A generalized characterization of algorithmic probability. [doi] [arxiv]
Theory of Computing Systems 61(4): 1337-1352.
In this technical paper I employ a fixed-point argument to show that the definition of algorithmic probability does not essentially rely on the uniform measure. A motivation for establishing this result was to question the view that algorithmic probability incorporates principles of indifference and simplicity.
- E.R.G. Quaeghebeur, C.C. Wesseling, E.M.A.L. Beauxis-Aussalet, T. Piovesan, and T.F.S. (2017): The CWI world cup competition: Eliciting sets of acceptable gambles. [pdf] [poster] Proceedings of Machine Learning Research 62 (ISIPTA 2017): 277-288. Poster presented at ISIPTA 2015.
-
T.F.S. (2016):
Solomonoff prediction and Occam's razor. [doi] [philsci]
Philosophy of Science 83(4): 459-479.Many writings on the subject suggest that Solomonoff's theory of prediction can offer a formal justification of Occam's razor. In this paper I make this argument precise and show why it does not succeed.
-
G. Barmpalias and T.F.S. (2011):
On the number of infinite sequences with trivial initial segment complexity. [doi] [preprint]
Theoretical Computer Science 412(52): 7133-7146.In this technical paper, based on results from my MSc thesis [pdf], we answer an open problem [pdf] in the field of algorithmic randomness. This problems concerns infinite sequences of minimal Kolmogorov-complexity. On the way we prove several results on the complexity of trees.
Invited publications
-
T.F.S. (2024):
Review of J.D. Norton, The Material Theory of Induction. [doi]
Philosophy of Science 91(4): 1030-1033. -
T.F.S. (2023):
Commentary on David Watson, "On the philosophy of unsupervised learning." [doi]
Philosophy & Technology 36,63:1-5 . -
T.F.S. (2020):
Review of D.G. Mayo: Statistical Inference as Severe Testing: How to Get Beyond the Statistics Wars. [doi]
Journal for General Philosophy of Science 51(3): 507-510.
-
T.F.S. (2018):
What's hot in mathematical philosophy. [pdf]
The Reasoner 12(12): 97-98.
-
J.-W. Romeijn, T.F.S. and P.D. Grünwald (2012):
Good listeners, wise crowds, and parasitic experts. [doi] [pdf]
Analyse & Kritik 34(2), pp. 399-408.
PhD dissertation (2018, cum laude)
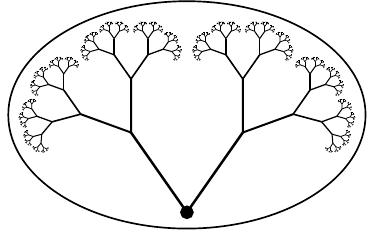
-
Universal prediction: A philosophical investigation. [cwi-repo] [handle] [philsci]
Supervisors: J.-W. Romeijn (U Groningen) and P.D. Grünwald (Centrum Wiskunde & Informatica, Amsterdam; Leiden U) .
Assessment committee: H. Leitgeb (LMU Munich), A.J.M. Peijnenburg (U Groningen), and S.L. Zabell (Northwestern U).
Examining committee: the assessment committee, and R. Verbrugge (U Groningen), L. Henderson (U Groningen), and W.M. Koolen (CWI Amsterdam).
In this thesis I investigate the theoretical possibility of a universal method of prediction. A prediction method is universal if it is always able to learn what there is to learn from data: if it is always able to extrapolate given data about past observations to maximally successful predictions about future observations. The context of this investigation is the broader philosophical question into the possibility of a formal specification of inductive or scientific reasoning, a question that also touches on modern-day speculation about a fully automatized data-driven science.
I investigate, in particular, a specific mathematical definition of a universal prediction method, that goes back to the early days of artificial intelligence and that has a direct line to modern developments in machine learning. This definition essentially aims to combine all possible prediction algorithms. An alternative interpretation is that this definition formalizes the idea that learning from data is equivalent to compressing data. In this guise, the definition is often presented as an implementation and even as a justification of Occam's razor, the principle that we should look for simple explanations.
The conclusions of my investigation are negative. I show that the proposed definition cannot be interpreted as a universal prediction method, as turns out to be exposed by a mathematical argument that it was actually intended to overcome. Moreover, I show that the suggested justification of Occam's razor does not work, and I argue that the relevant notion of simplicity as compressibility is problematic itself.
- My thesis was one of the three winners of the triennial Wolfgang Stegmüller Award of the Gesellschaft für Analytische Philosophie. Here is a picture from the ceremony, taken from this slideshow.